Leela Chess Zero for Peak Performance
Introduction
The landscape of computer chess has been irrevocably transformed by the emergence of neural-network-based engines, and at the forefront of this revolution stands Leela Chess Zero (Lc0). Born as an open-source implementation inspired by Google’s AlphaZero, Leela Chess Zero harnesses the power of deep learning and Monte Carlo Tree Search (MCTS) to evaluate positions and select moves. Unlike traditional engines such as Stockfish, which rely extensively on brute-force search and handcrafted evaluation functions, Lc0 integrates a trained neural network to guide its search, leading to a fundamentally different approach to chess-playing strength and style.
This tutorial aims to provide a comprehensive guide on configuring and utilising Leela Chess Zero in the most efficient and powerful manner possible. We will cover the complete process of installation and setup, from downloading the engine to integrating it within a chess GUI, drawing upon official resources such as the Lc0 Quickstart and Download pages. Further, we will delve into detailed configuration options via both UCI parameters and command-line flags, ensuring that users can tailor the engine to their hardware and preferences. Beyond software configuration, we will explore the hardware requirements necessary to achieve performance on par with the leading conventional engine Stockfish, discussing GPU and CPU specifications, as well as practical recommendations for achieving optimal knps (kilo nodes per second) rates.
In addition to setup and hardware considerations, this article will highlight Lc0’s most significant achievements in computer chess, including landmark victories in the Top Chess Engine Championship (TCEC) and World Computer Chess Championship (WCCC), as well as its Elo performance trends over time. By examining these milestones, readers will gain insight into how neural-network approaches have challenged and, in many instances, outperformed traditional engines.
Finally, we will conclude with a forward-looking perspective on the prospects of the Leela Chess Zero project. We will analyse whether Lc0 has the potential to claim the top spot in the world rankings of chess engines, considering ongoing developments in network architectures, training strategies, and hardware advancements. By the end of this article, readers will possess not only the practical know-how to configure Lc0 optimally but also a deep appreciation of its place within the evolving ecosystem of computer chess.
Installation and Setup
Setting up Leela Chess Zero correctly is the critical first step toward harnessing its full potential. The process begins by selecting an appropriate chess graphical user interface (GUI), as Lc0 communicates exclusively via the UCI (Universal Chess Interface) protocol. Popular free GUIs such as Arena, Cutechess, and BanksiaGUI support UCI engines and include convenient options for adding external engines like Lc0. Commercial alternatives like ChessBase also offer UCI compatibility, though they require a licensed purchase. Guidance for GUI-specific integration can be found in the official documentation on the Lc0 website (lczero.org).
Once a GUI has been chosen, the next step is to download the Lc0 engine binary. The official Download page provides platform-specific packages for Windows, macOS (via Homebrew), Android, and source code for custom builds (lczero.org). Windows users should select the version that matches their GPU architecture, such as CUDA for modern NVIDIA cards or OpenBLAS for CPU-only systems. macOS users can execute brew install lc0
, which installs both the engine and a default neural network. Android users receive a BLAS-based build that runs on mobile hardware.
After downloading, extract the contents of the archive into a dedicated directory of your choice. Key files include:
- lc0.exe (or equivalent binary): The engine executable.
*.pb.gz
** network file**: The default neural network used for position evaluation.- DLLs or shared libraries: Required dependencies for GPU acceleration or other backends.
- lc0-training-client.exe: A separate client for contributing self‑play games to the training pipeline, not needed for gameplay.
Ensure that the network file is co‑located with the engine binary. If multiple network files exist, Lc0 will automatically select the most recently modified one by default (lczero.org).
With the engine and network in place, open your chosen GUI and navigate to the engine management section (often labelled “Add Engine” or similar). Create a new engine entry, assigning a name such as “Leela Chess Zero” and pointing the executable path to lc0.exe
. Most GUIs will automatically detect UCI‑compatible parameters and allow modification of common settings like threads and hash size.
At this point, you should be able to initiate analysis or play games against Lc0 directly within the GUI. For advanced users, further configuration can be performed through UCI options or command‑line flags, which we will explore in the next section.
Configuration Options
Leela Chess Zero offers extensive configuration capabilities to tailor both its neural‑network evaluation and search behaviour. Three primary methods exist for setting parameters:
- UCI Parameters: Exposed via the GUI, these settings allow on‑the‑fly adjustment of critical options such as
Threads
,NNCacheSize
, andSyzygyPath
. GUIs such as Cutechess present a dedicated UCI options dialog, enabling users to modify values without restarting the engine (lczero.org). - Command Line Flags: For users who prefer launching Lc0 outside a GUI or need to specify low‑level options, flags passed directly to
lc0.exe
(e.g.,--backend=cudnn-auto
,--minibatch-size=256
) grant full control. Executinglc0.exe --help
lists all available flags and their default values (lczero.org). - Configuration File: To manage complex setups or multiple distinct configurations, place a text file named
lc0.config
(or any custom name passed via the--config
flag) in the engine directory. Each line contains one flag (with or without leading--
), allowing batch application of settings such as:weights=C:\Networks\supernova.pb.gz cpuct-at-root=3.0 syzygy-fast-play=true
This approach streamlines experimental tuning without manual GUI adjustments.
Key parameters of particular interest include:
- Backend Selection (
--backend
): Determines the neural‑network computation engine (e.g.,cudnn-auto
,onnx-dml
,dnnl-blas
) optimized for your hardware. - Threads (
--threads
): Number of CPU worker threads, typically matching the number of physical cores for optimal parallel search. - NNCacheSize (
--nncache
): Size of the neural‑network evaluation cache; larger caches improve reuse but consume more RAM. - MinibatchSize (
--minibatch-size
): Batch size for GPU inference; balance strength and speed by testing values between 64 and 512. - CPuct and CPuctAtRoot: UCT constants influencing exploration vs. exploitation in MCTS; modest adjustments here can alter playing style.
- TimeManager (
--time-manager
): Choose betweenlegacy
,smooth
, oralphazero
time managers; thealphazero
manager budgets 5% of remaining time per move by default (lczero.org).
By combining these configuration methods, users can fine‑tune Lc0 to their preferences, balancing strength, style, and resource consumption.
Hardware Requirements
Leela Chess Zero’s reliance on neural‑network inference means that GPU performance becomes the critical factor in determining strength, in contrast to traditional engines that primarily depend on raw CPU speed and transposition‑table size. To achieve performance comparable to a leading Stockfish configuration—commonly running on 16–32 CPU threads with large hash tables—Lc0 users should prioritise high‑end NVIDIA GPUs.
Practical benchmarks indicate the following equivalences:
- NVIDIA GeForce RTX 2080 Ti: Approximately on par with a 16‑core CPU in terms of overall playing strength, assuming default networks and typical settings (chess.com).
- NVIDIA GeForce RTX 4090: Delivers roughly double the knps of a 3080 Ti, achieving ~50 knps on the standard 512×20 network, mirroring the throughput of 2× A100 GPUs used in TCEC Season 15 (lichess.org).
- 2× NVIDIA A100 (TCEC Standard): Provides the ultimate benchmark, reaching ~50 knps on mid‑sized networks, although modern consumer GPUs like the 4090 can approximate this performance for a fraction of the cost.
For users without access to a dedicated GPU, Lc0 supports CPU‑only backends (dnnl-blas
, openblas
), but performance drops sharply, often yielding strength several hundred Elo points lower than an equivalently priced GPU setup.
When comparing cost‑effectiveness, consider that a high‑end GPU such as the RTX 4090 (retail around £1,600) can match or exceed the performance of dual $3,000+ CPUs and hundreds of gigabytes of RAM required for a top‑tier Stockfish setup. Furthermore, energy efficiency per position evaluated tends to favour GPUs, providing longer running times for analysisServer tasks on lower power draw relative to equivalent CPU performance.
Additional hardware considerations:
- CPU: While Lc0 offloads most computations to the GPU, a multi‑core CPU remains necessary to handle UCI communication, batch preparation, and other auxiliary tasks. A modern 8‑core CPU with high single‑thread performance is sufficient for most configurations.
- RAM: Neural network caches and transposition tables require memory; 16–32 GB of system RAM is recommended to accommodate both GPU and CPU‑side caches comfortably.
- Storage: Fast SSD storage accelerates network loading and reduces GUI lag when switching weights or reviewing large databases.
By aligning hardware choices with the engine’s architecture, users can achieve playing strength on par with, or even exceeding, traditional engines such as Stockfish, provided they invest in appropriate GPU resources.

Key Achievements of Leela Chess Zero
Since its inception in early 2018, Leela Chess Zero has amassed a series of landmark achievements that underscore its rapid ascent in computer chess. Within months of its launch, the engine demonstrated grandmaster‑level play, surpassing early versions of established engines such as Rybka and Komodo despite evaluating orders of magnitude fewer positions per second (en.wikipedia.org).
World Computer Chess Championship (WCCC) 2019
In April 2019, Lc0 secured a historic victory at the World Computer Chess Championship, becoming the first neural‑network–based engine to win the event against a field dominated by traditional minimax engines (en.wikipedia.org). This triumph validated the efficacy of reinforcement‑learning techniques and MCTS in chess, marking a paradigm shift in engine design.
Top Chess Engine Championship (TCEC) Superfinals
- Season 15 (2019): Lc0 qualified for the superfinal after undefeated performance in Division P, ultimately defeating Stockfish (+14 =79 -7) in a 100‑game match to claim the TCEC title (chessprogramming.org). This victory underscored Lc0’s capability to compete at the highest level under professional time controls.
- Subsequent Seasons: While Stockfish reclaimed the title in Seasons 16 through 20, Leela Chess Zero demonstrated continued progress, finishing as runner‑up in multiple seasons and achieving title defence victories in later seasons such as Season 17 (+17 =71 -12) and Season 23 (+27 =63 -10) (de.wikipedia.org).
Elo Progression and Self‑Play Training
Leela Chess Zero’s Elo rating has climbed steadily over time, driven by a self‑play distributed training framework. As of 2024, Lc0 has played over 2.5 billion games against itself, learning from approximately 1 million daily self‑play games and continually refining its neural networks (en.wikipedia.org). This volume of data has enabled the engine to incorporate complex positional understanding, endgame tablebase knowledge, and even emergent tactics typically associated with human strategic thinking.
Spinoffs and Algorithmic Innovations
Beyond its own development, Lc0 has spawned derivative projects and inspired novel search algorithms:
- AllieStein: A hybrid engine that combines Lc0’s neural networks with a bespoke search algorithm, achieving competitive placements in TCEC Seasons 15 and beyond.
- Ceres: A C# port introducing algorithmic improvements and participating successfully in Swiss and cup formats.
- Transformer‑Based Networks: Recent network architectures have transitioned from residual blocks to transformer‑style models, further enhancing evaluation efficiency and positional insight.
Together, these achievements attest to Lc0’s transformative impact on computer chess, elevating neural‑network approaches from experimental novelties to world‑beating contenders.
Future Prospects and Conclusion
Leela Chess Zero’s journey from an experimental AlphaZero clone to a premier chess engine has been nothing short of remarkable. Its success in major championships, sustained Elo gains, and widespread community adoption illustrate the viability of neural‑network–driven search. Looking ahead, several factors will determine whether Lc0 can unseat Stockfish and claim permanent dominance at the top of engine rankings:
- Network Architecture Advancements: Continuing innovation in neural‑network design—such as more efficient transformer models and quantized weights—will enable deeper positional understanding and reduced inference latency.
- Training Data Quality and Quantity: Expanding the volume of diverse self‑play games, incorporating specialised endgame training, and integrating supervised learning from human and engine master games will enrich the training corpus.
- Hardware Evolution: As GPU technology advances—particularly with the rollout of next‑generation tensor‑core architectures—Lc0 will benefit from accelerated inference speeds, allowing larger networks to be used in real‑time analysis and over-the-board play.
- Search Algorithm Enhancements: While MCTS remains central to Lc0’s strategy, hybrid approaches that combine neural guidance with traditional pruning techniques (e.g., smart pruning, dynamic time management) may yield further strength gains.
- Community and Development Momentum: The open‑source nature of the project fosters rapid iteration, volunteer contributions, and benchmarking, ensuring that Lc0 remains at the cutting edge of engine development.
Given these dynamics, Lc0 is well‑positioned to challenge—and potentially surpass—Stockfish in the coming years. Already, specialized deep‑learning accelerators and cloud‑based training have demonstrated promising results. As the gap between network evaluation speed and GPU capabilities narrows, Lc0’s unique style and strategic depth may tip the balance.
In conclusion, configuring Leela Chess Zero for optimal performance requires careful attention to installation, network selection, and engine parameters, all while leveraging appropriate GPU hardware. The engine’s landmark successes in premier championships, coupled with ongoing advances in neural‑network research, suggest that Lc0’s trajectory remains firmly upward. Whether you are a casual enthusiast seeking the most human‑like analysis or a professional competitor requiring the cutting‑edge engine, Leela Chess Zero offers a compelling fusion of art and algorithm—one that stands poised to shape the future of computer chess.
Bibliography
- “Getting Started”. Leela Chess Zero Quickstart page. (lczero.org)
- “Download Lc0”. Leela Chess Zero Download page. (lczero.org)
- “Engine parameters”. Leela Chess Zero Flags page. (lczero.org)
- “Time management”. Leela Chess Zero Configuration page. (lczero.org)
- Reddit: “Best Hardware for Leela (Lc0 Alpha Zero) in 2023”. Lichess forum. (lichess.org)
- “Leela Chess Zero”. Wikipedia. (en.wikipedia.org)
- “TCEC Season 15”. Wikipedia. (en.wikipedia.org)
- ChessProgramming.org: “Leela Chess Zero” achievements. (chessprogramming.org)
- Top Chess Engine Championship results. Wikipedia. (de.wikipedia.org)
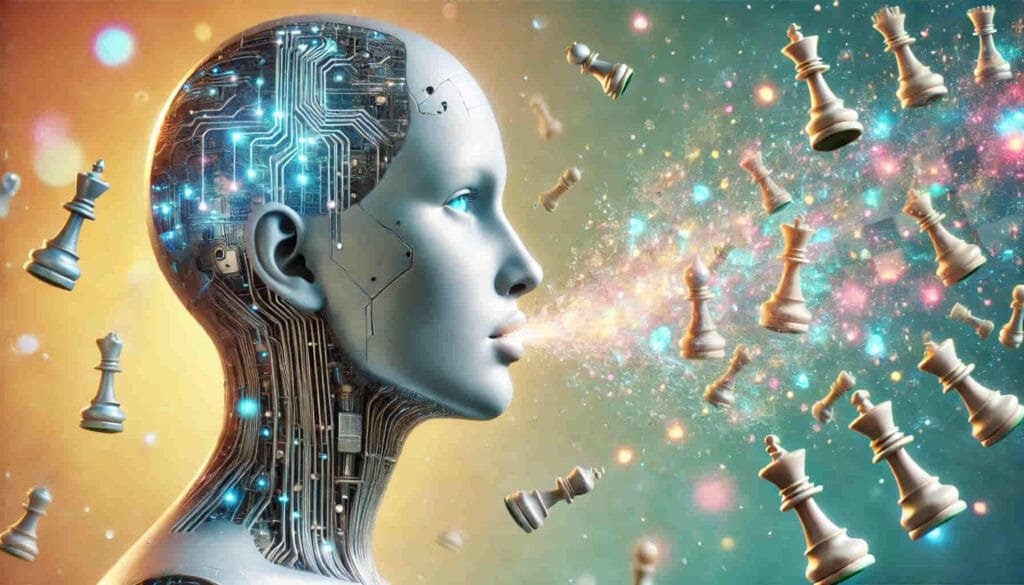
Jorge Ruiz Centelles
Filólogo y amante de la antropología social africana