Experience File in a UCI Chess Engine
Creating an experience file for a UCI chess engine is an advanced technique that allows the engine to learn from past games and improve its decision-making process. In modern chess programming, experience files serve as repositories of historical data including moves, evaluations, and outcomes of previous games. By utilizing these files, engines can adjust their strategies, learn from their mistakes, and optimize their performance in subsequent games. This article is based on the reference guide available at deeds.mynetgear.com, and it covers the sections on Experiments, Experience Files, Learning Quiz, and Learning Sessions. In addition to the material provided in the reference, this article expands on the process with further insights and technical details to help developers and chess enthusiasts create robust experience files from scratch.
The following sections detail the methodology, from the initial experiments for data collection to the integration of learning sessions that allow the chess engine to harness the full potential of its historical data. Whether you are working with engines such as Eman, HypnoS, or BrainLearn (which use experience files) or exploring advanced techniques in chess AI, this comprehensive guide will equip you with the knowledge necessary to implement and optimize experience files effectively.
Introduction
Experience files in UCI chess engines are a cornerstone of modern chess AI development. They are essentially databases that log a detailed history of games played by the engine. This includes every move made, the evaluation of those moves, the final outcome of each game, and even the statistical performance of different strategies. The primary objective behind creating these files is to provide a mechanism for the engine to “learn” from its past performance, thereby continuously refining its approach to gameplay.
To fully appreciate the importance of experience files, it is essential to understand that traditional chess engines rely heavily on brute-force computation and evaluation functions to choose their moves. However, as the complexity of chess increases, and with the advent of machine learning techniques, simply calculating moves is no longer sufficient for achieving top-tier performance. Experience files allow engines to analyze trends, recognize patterns, and adapt strategies based on historical data. This not only results in more efficient gameplay but also helps in reducing repeated mistakes.
In the context of UCI (Universal Chess Interface) engines, the integration of an experience file represents a significant step towards developing a more “human-like” learning process. By continuously updating the experience file with data from new games, the engine can identify which strategies lead to success and which result in poor outcomes. This feedback loop is analogous to how human players learn from their experiences over time, gradually improving their skills through practice and analysis.
Key components of an experience file include:
- Move Records: Detailed logs of every move played, including the starting position and the resulting board state.
- Evaluation Scores: Numerical evaluations associated with each move, which indicate the potential advantage or disadvantage of the move.
- Game Outcomes: Final results of the games (win, loss, or draw), providing a clear metric for evaluating the success of specific strategies.
- Statistical Data: Aggregated data that highlights recurring patterns, error rates, and performance trends over time.
The development and utilization of experience files also have implications for the broader field of artificial intelligence. By enabling the engine to “remember” past games, developers can apply reinforcement learning techniques to further improve the engine’s decision-making processes. This synergy between traditional rule-based approaches and modern AI techniques leads to engines that are not only powerful in calculation but also adaptive and strategic.
Furthermore, the reference guide at deeds.mynetgear.com provides a structured approach to creating these files, emphasizing the importance of detailed experiments, rigorous data collection, and systematic learning sessions. This article will explore each of these components in depth, beginning with the experiments designed to gather relevant data, moving through the creation and management of the experience files, and concluding with the integration of learning sessions that allow the engine to utilize this historical data to enhance its performance.
In the following sections, we will explore each phase of the process with comprehensive detail, ensuring that every aspect—from the initial experimental setup to the final configuration of the learning sessions—is clearly explained and supported by best practices in chess programming. By the end of this article, you will have a clear understanding of how to build an experience file from scratch and effectively integrate it with your UCI chess engine, paving the way for a smarter, more adaptable chess AI.
Experiments
The experiments section of the guide on deeds.mynetgear.com lays the foundation for creating an effective experience file. This phase is critical because it involves systematically collecting the data that the engine will later use to learn and improve. The experiments are designed to simulate a variety of gameplay conditions and record the corresponding outcomes and evaluations.
Detailed Overview of Experiments
The experiments process involves several key steps, each carefully designed to ensure that the data collected is comprehensive and useful for the experience file. This process includes the following phases:
- Setting Up the Test Environment:
- Selecting the Appropriate UCI Engine:
Choose a chess engine that supports experience file integration. While engines like AlphaZero or LeelaChess do not use experience files, others such as Eman, HypnoS, and BrainLearn do. The selection should be based on the specific requirements of your project. - Environment Configuration:
Establish a robust testing environment that includes both a graphical user interface (GUI) and any auxiliary software necessary to facilitate the experiment. This environment should be stable and capable of handling long-duration tests without interruptions. - Parameter Initialization:
Define the key parameters that will govern the experiments. This includes the search depth, time allocated per move, and any other engine-specific settings that influence gameplay. It is crucial to standardize these parameters to ensure consistency across experiments.
- Selecting the Appropriate UCI Engine:
- Running Test Games:
- Defining Experiment Protocols:
Develop a set of protocols for running test games. This includes rules for when to reset the engine, how to handle draw scenarios, and the method of recording every move. A well-documented protocol is essential for reproducibility. - Data Collection:
During each game, every move is logged along with its evaluation score. The data must capture not just the move itself but also the context in which it was played, such as the board state and any notable deviations from optimal play. - Handling Variability:
To ensure that the data reflects a wide range of scenarios, introduce variability in the game conditions. This might include testing under different time controls, varying levels of opponent strength, or even experimenting with non-standard openings. The goal is to expose the engine to a diverse set of situations so that the experience file becomes a rich repository of knowledge.
- Defining Experiment Protocols:
- Analyzing Experiment Data:
- Data Cleaning:
Once the games have been played, the raw data must be cleaned and organized. This involves filtering out any incomplete records or anomalies that may skew the analysis. - Pattern Identification:
Use statistical tools to identify patterns in the data. For instance, analyze which moves consistently lead to advantageous positions and which patterns correlate with wins or losses. This analysis is pivotal for later stages of learning. - Performance Metrics:
Evaluate the engine’s performance using several metrics, including win/loss ratios, average move quality, and response times. These metrics provide quantitative feedback on the engine’s strengths and weaknesses.
- Data Cleaning:
Additional Considerations and Best Practices
Data Integrity:
Ensuring that your experiment data is accurate and complete is non-negotiable. Implementing rigorous logging protocols and cross-checking results with manual reviews can significantly enhance data integrity.
Automation:
Where possible, automate the experimental process. Scripts and automated testing frameworks can help run a large number of games with minimal human intervention, thereby speeding up the data collection process.
Documentation:
Keep detailed records of every experiment, including the configuration parameters, any deviations from the standard protocol, and observational notes. This documentation is invaluable for troubleshooting and refining your approach in future experiments.
Feedback Loop:
Incorporate a feedback loop in the experimental design. As you analyze the data, continuously refine your test conditions to target specific aspects of engine performance. This iterative process is key to developing an effective experience file.
Expected Results and Their Impact
The experiments yield a wealth of data, including:
- Move-by-Move Analysis:
Every move is documented with corresponding evaluation scores, providing insights into the engine’s decision-making process. - Outcome Statistics:
Aggregated statistics such as win percentages, draw frequencies, and loss ratios offer a quantitative measure of the engine’s overall performance. - Trend Identification:
Patterns in the data reveal which strategies are most effective, helping developers understand the strengths and weaknesses of the engine. - Error Analysis:
By identifying moves that consistently lead to suboptimal outcomes, developers can target specific areas for improvement.
The thorough experimental process not only provides the raw data needed to create an experience file but also lays the groundwork for ongoing engine improvement. With each experiment, the engine’s performance data becomes richer, enabling more precise adjustments and refinements in later learning sessions.
By following these detailed experimental protocols, you ensure that the data captured is robust and comprehensive. This, in turn, forms the cornerstone of the experience file, a critical tool that will drive your engine’s learning and evolution. The insights gleaned from this phase are essential for all subsequent steps, from formatting the experience file to integrating it with the engine during learning sessions.
Experience Files
The experience files are the repositories where all the experimental data is stored in an organized, accessible format. They serve as the historical memory of the chess engine, allowing it to analyze previous moves, evaluate the outcomes, and adjust future strategies accordingly. The section on Experience Files in deeds.mynetgear.com provides a detailed explanation of how to create and manage these files effectively.
In-Depth Look at Experience Files
Experience files must be meticulously structured to ensure that they capture all relevant data while remaining easily accessible for the engine during gameplay. Here are the core components and best practices involved in creating an effective experience file:
- Data Formatting and Structure:
- Standardized Formats:
The data gathered from experiments should be formatted in a standardized way. Common formats include CSV, JSON, or even custom binary formats specifically designed for high-performance reading and writing. Standardization helps in ensuring consistency and facilitates easy integration with the chess engine. - Hierarchical Organization:
Organize the data hierarchically so that it is easy to navigate. For instance, you can group data by game, then by move, and within each move, include the evaluation score and additional metadata such as board position, timestamp, and any special conditions. - Data Compression:
Given the potentially vast amount of data generated during experiments, implementing compression techniques can help save disk space and speed up access times. Techniques such as gzip compression or other custom algorithms can be useful.
- Standardized Formats:
- Storing and Managing Data:
- Efficient Storage Solutions:
Choose storage solutions that allow for rapid access and update. Relational databases, NoSQL solutions, or even dedicated file systems can be employed based on the scale of data and performance requirements. - Data Integrity Checks:
Implement routines to verify data integrity. This includes periodic checks to ensure that no records are corrupted and that the data remains consistent over time. Regular backups are also critical to prevent data loss. - Metadata Inclusion:
Alongside the move data, include metadata such as the date and time of each game, the parameters used during the experiment, and version information for the engine. This metadata is invaluable for tracking progress and understanding changes in performance over time.
- Efficient Storage Solutions:
- Integration with the UCI Engine:
- File Accessibility:
Ensure that the experience file is placed in a location that is both accessible to and writable by the chess engine. The engine’s configuration should point to the exact path where the experience file is stored. - Reading and Writing Protocols:
Develop robust protocols for reading from and writing to the experience file. The engine should be able to quickly update the file after every game and retrieve data in real time during gameplay. This requires efficient file I/O operations and possibly even caching mechanisms. - Error Handling:
Build in comprehensive error handling routines to manage any issues that arise from file corruption or access errors. The engine should be able to gracefully handle such errors without interrupting gameplay.
- File Accessibility:
Benefits of Using Experience Files
The primary benefits of well-structured experience files include:
- Enhanced Decision-Making:
With access to a vast repository of historical data, the engine can make more informed decisions. By analyzing previous moves and their outcomes, the engine can better predict which moves are likely to lead to success. - Strategic Optimization:
Experience files enable the engine to recognize recurring patterns and strategies that consistently yield positive results. This facilitates the refinement of the engine’s strategic approach over time. - Error Minimization:
By learning from past mistakes documented in the experience file, the engine can adjust its evaluations and reduce the frequency of suboptimal moves. - Continuous Learning:
The integration of experience files creates a feedback loop that is essential for continuous improvement. The engine continuously updates its database, which in turn informs future gameplay strategies.
Additional Insights
Integration with AI and Machine Learning:
Beyond traditional data logging, integrating machine learning techniques with experience files can further enhance performance. Techniques such as reinforcement learning or deep learning can be used to analyze the experience file and identify subtle patterns that are not immediately obvious through traditional statistical analysis.
Scalability and Future-Proofing:
As the number of games increases, the experience file can become extremely large. Planning for scalability from the outset—by choosing appropriate data structures and storage solutions—ensures that the system remains responsive even as the dataset grows.
Security Considerations:
If the experience file is being used in competitive environments or in cloud-based systems, ensure that proper security measures are in place. This might include encryption of the data at rest and during transmission, as well as access controls to prevent unauthorized modifications.
By following these best practices, you ensure that the experience file not only serves as an effective learning tool but also as a reliable and efficient repository of data. This section of the guide provides the technical backbone for building a robust experience file that significantly enhances the engine’s overall performance.
Learning Quiz
The Learning Quiz is an integral part of the guide on deeds.mynetgear.com. It is designed to test your understanding of the concepts involved in creating and using an experience file for a UCI chess engine. The quiz not only reinforces learning but also provides immediate feedback on key concepts that are essential for the successful integration of an experience file into the engine.
Detailed Examination of the Learning Quiz
The Learning Quiz comprises a series of questions that cover various aspects of the process—from the basic definition of an experience file to the specifics of data integration and engine configuration. Each question is designed to challenge your understanding and ensure that you have grasped the critical elements required for implementing an experience file effectively.
Sample Quiz Questions and Their Explanations
- What is an experience file in a UCI chess engine?
- Correct Answer:
An experience file is a repository that stores detailed information about games played, including moves, evaluations, and outcomes, which the engine uses to improve its performance. - Explanation:
This answer is correct because the primary purpose of an experience file is to provide a historical record that the engine can analyze to learn from past performances. This data-driven approach is fundamental to enhancing the engine’s decision-making process.
- Correct Answer:
- What is the main benefit of using an experience file?
- Correct Answer:
The main benefit is the enhancement of the engine’s decision-making capabilities by learning from past games and adjusting strategies accordingly. - Explanation:
Experience files enable the engine to identify recurring patterns and avoid repeated mistakes, leading to more effective gameplay. This iterative learning process is what sets experience-based systems apart from purely computational engines.
- Correct Answer:
- What types of data are typically stored in an experience file?
- Correct Answer:
The file typically includes move records, evaluation scores for those moves, game outcomes, and associated metadata. - Explanation:
This data is critical because it not only captures the sequence of moves but also provides quantitative assessments of their effectiveness. This comprehensive dataset forms the basis for the engine’s strategic improvements.
- Correct Answer:
- How does an experience file contribute to error reduction in gameplay?
- Correct Answer:
By analyzing historical data, the engine can identify moves that consistently lead to suboptimal outcomes, allowing it to adjust its decision-making process and avoid similar mistakes in future games. - Explanation:
The ability to learn from previous errors is a core advantage of using an experience file. This continuous feedback loop significantly reduces the likelihood of repeated mistakes, thus enhancing overall performance.
- Correct Answer:
Importance of the Learning Quiz
The Learning Quiz is not merely a set of questions; it is a learning tool in itself. It helps ensure that:
- Fundamental Concepts Are Understood:
By answering quiz questions, users can confirm that they understand the underlying principles of experience file creation and integration. - Practical Application Is Reinforced:
The quiz forces you to think about how each aspect of the experience file fits into the overall learning framework of the chess engine. - Immediate Feedback Leads to Improvement:
With detailed explanations provided for each question, users can identify areas where they need further study and adjust their approach accordingly.
Further Insights and Best Practices
Iterative Learning:
The Learning Quiz should be used as an iterative process. As you update your experience file and refine your experimental data, revisit the quiz to ensure that your understanding remains aligned with the evolving methodology.
Customized Quizzes:
For advanced developers, consider creating custom quizzes that reflect the unique aspects of your experimental setup and data collection methods. This customization can further enhance the learning experience by addressing specific challenges encountered in your project.
Integration with Learning Sessions:
Link the results of the Learning Quiz with the Learning Sessions phase. The quiz results can inform which areas of the experience file need further refinement and which parameters might require adjustment in the learning sessions.
In summary, the Learning Quiz is a crucial step that consolidates theoretical knowledge with practical application. It ensures that every detail of the process is well understood before proceeding to the more complex phase of integrating the experience file with the engine during learning sessions. This thorough understanding is vital for achieving a high-performing chess engine that continuously evolves and improves.
Learning Sessions
Learning Sessions represent the final phase in the process of integrating an experience file into a UCI chess engine. During these sessions, the engine utilizes the historical data stored in the experience file to engage in continuous learning, refining its strategies based on past performance. The guide on deeds.mynetgear.com offers a detailed explanation of how to configure and execute these sessions, and this section expands on those guidelines with additional technical details and best practices.
Comprehensive Guide to Learning Sessions
Learning Sessions are structured training periods where the chess engine actively analyzes its experience file and updates its internal evaluation parameters accordingly. This phase is essential for transforming static data into dynamic learning, resulting in improved gameplay over time.
- Preparing the Experience File:
- Verification of Format:
Before initiating a Learning Session, verify that the experience file is correctly formatted and free of errors. The file must be in a standardized format (e.g., JSON, CSV) that the engine can parse without issues. - File Location and Accessibility:
Ensure that the file is stored in a location that the engine can easily access. Update the engine’s configuration to point to the file’s path, and test the file’s accessibility before starting the session. - Data Integrity and Backup:
Perform integrity checks to ensure that the data is complete and accurate. Regular backups should be made to avoid any loss of critical data during the learning process.
- Verification of Format:
- Configuring the UCI Engine for Learning:
- Specifying the Experience File:
In the engine’s settings, specify the location of the experience file. This might involve editing configuration files or using command-line parameters to ensure that the engine loads the correct data. - Adjusting Learning Parameters:
Define parameters such as search depth, time per move, and learning rate. These parameters determine how the engine will weigh historical data against real-time analysis during the session. - Enabling Real-Time Updates:
Configure the engine to update the experience file in real time. This ensures that every new game contributes to the learning process, creating a continuously evolving database of moves and evaluations.
- Specifying the Experience File:
- Executing the Learning Sessions:
- Starting the Session:
Initiate the Learning Session by allowing the engine to play a series of games or simulated matches using the experience file. Monitor the engine’s performance and ensure that it is correctly referencing the file throughout the session. - Monitoring Progress:
Track key performance indicators such as move accuracy, win/loss ratios, and decision times. Regularly review logs and output data to assess whether the engine is learning from its past experiences. - Iterative Adjustments:
Based on performance data, adjust learning parameters as needed. For example, if the engine is overfitting to past moves, reduce the weight given to historical data or increase the randomness in move selection. - Integration with External Tools:
Consider using external analysis tools that can visualize the evolution of the engine’s performance over multiple sessions. Graphical representations of data trends can provide valuable insights into how well the learning process is progressing.
- Starting the Session:
Advantages and Expected Outcomes
The successful execution of Learning Sessions results in several notable improvements in the engine’s performance:
- Adaptive Strategies:
The engine develops more adaptive strategies by continuously learning from a growing repository of historical data. - Refined Decision-Making:
By analyzing patterns from previous games, the engine can better predict which moves will lead to successful outcomes and avoid those that have historically resulted in mistakes. - Performance Optimization:
Continuous adjustments based on real-time feedback lead to a more efficient search process, reduced error rates, and improved overall performance.
Additional Considerations for Advanced Users
Combining with Machine Learning Algorithms:
For developers seeking to push the boundaries of chess AI, integrating machine learning algorithms into the Learning Sessions can further enhance the process. Techniques such as reinforcement learning, neural network evaluations, and even unsupervised learning can be incorporated to analyze complex patterns in the experience file.
Multi-Session Learning:
In advanced configurations, consider organizing Learning Sessions into multiple phases. Each phase can focus on a different aspect of gameplay—opening strategies, mid-game tactics, or endgame scenarios. This modular approach allows for more targeted learning and easier troubleshooting of specific issues.
Cross-Engine Comparisons:
Experiment with using the same experience file across different UCI engines that support experience-based learning. Comparing the performance improvements of engines like Eman, HypnoS, and BrainLearn can provide insights into the relative effectiveness of different learning algorithms and data structures.
By meticulously configuring and executing Learning Sessions, you ensure that the chess engine is not only using its historical data effectively but also evolving in a way that maximizes its competitive performance. This final phase of integration transforms raw experimental data into actionable intelligence that continuously enhances the engine’s capabilities.
Conclusion
In conclusion, creating an experience file for a UCI chess engine is a multi-step process that integrates data collection, meticulous file management, and advanced learning techniques to produce a self-improving chess AI. The methodology outlined in this article—based on the comprehensive guide available at deeds.mynetgear.com—provides a robust framework for collecting game data, structuring it into an experience file, and leveraging that file during dedicated Learning Sessions to optimize engine performance.
Recap of the Methodology
- Data Collection Through Experiments:
Conduct rigorous experiments to gather detailed data on game moves, evaluations, and outcomes. This step lays the foundation for building a comprehensive experience file. - Creation and Management of Experience Files:
Organize and format the collected data into a standardized, accessible format. Ensure that the experience file is properly structured with hierarchical organization, data integrity checks, and efficient storage mechanisms. - Integration and Learning Sessions:
Configure the UCI chess engine to use the experience file by setting the correct parameters and file paths. Conduct Learning Sessions where the engine continuously updates its strategies based on historical data, leading to improved decision-making and performance optimization. - Continuous Improvement:
Utilize iterative feedback loops, adjust learning parameters, and possibly integrate machine learning techniques to further refine the engine’s performance over time. Advanced users may explore multi-phase learning sessions and cross-engine comparisons to push the boundaries of chess AI.
Final Thoughts
The creation of an experience file represents a significant advancement in the field of chess engine development. By meticulously documenting and analyzing past games, developers can build engines that not only calculate moves with precision but also adapt intelligently based on historical performance. This approach is essential in today’s competitive environment where artificial intelligence is continually evolving, and where the ability to learn from experience is a critical differentiator.
The methods discussed in this article provide a clear and comprehensive guide for anyone interested in enhancing a UCI chess engine through the integration of experience files. Whether you are a hobbyist programmer or a professional in the field, following these best practices will help you create a robust learning system that continuously evolves and adapts to new challenges.
Bibliography and Further Reading
- deeds.mynetgear.com: The primary reference for experiments, experience files, learning quiz, and learning sessions.
- UCI Protocol Documentation: For detailed technical specifications on how UCI engines communicate and integrate with external files.
- Chess Engine Programming Literature: Various academic and professional publications on AI, machine learning, and chess engine development.
- Reinforcement Learning Texts: To explore how modern AI techniques can complement traditional experience file methodologies.
By implementing the strategies detailed in this guide, you will be well-equipped to develop a UCI chess engine that not only performs at a high level but also continuously improves over time, making it a formidable opponent in any competitive setting.
This comprehensive article has provided an in-depth look at how to create and utilize an experience file in a UCI chess engine. By integrating experimental data, carefully managed experience files, iterative Learning Sessions, and continuous feedback loops, you can develop a chess engine that is both smart and adaptive. Whether you are working with engines like Eman, HypnoS, or BrainLearn, these methodologies offer a pathway to enhanced performance and strategic depth in chess AI development.
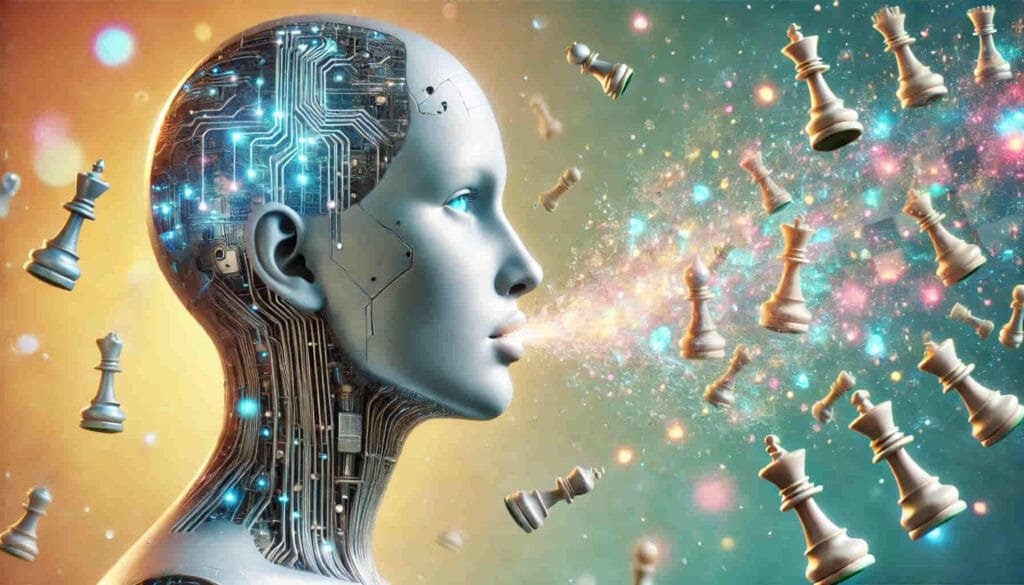
Jorge Ruiz Centelles
Filólogo y amante de la antropología social africana